Introduction:
In the world of artificial intelligence and machine learning, data is the fuel that powers decision-making processes. However, this data isn’t inherently usable—raw data must be categorized, labeled, and structured. That’s where Data Annotation Tech steps in. This essential technology transforms raw information into organized data, facilitating the accurate training of machine learning models and the overall success of AI-driven solutions.
Key Takeaways:
- Data Annotation Tech enables the structuring and labeling of large datasets for AI and machine learning models.
- This tech ensures high-quality training for algorithms, driving improvements in various industries.
- Key techniques include image, text, audio, and video annotation, each playing a crucial role in AI performance.
- Demand for skilled annotators and automated systems is on the rise due to the growing reliance on AI.
- Future innovations will likely focus on automation and improving annotation accuracy to handle even larger datasets.
What Is Data Annotation Tech?
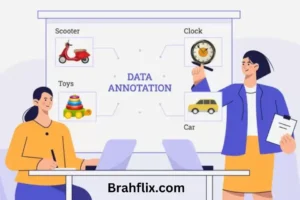
Data Annotation Tech refers to the suite of tools and methods used to label and organize data, making it suitable for machine learning algorithms. Annotations may include labeling objects in images, marking text for sentiment analysis, or tagging parts of a video for behavior detection. This process ensures that AI systems are trained on high-quality, structured datasets, ultimately increasing the accuracy of their predictions.
Key Point: Annotated data helps models recognize patterns, learn from them, and make accurate decisions in real-time.
Examples of Data Annotation:
- Image Annotation: Identifying objects like cars, people, or animals in images.
- Text Annotation: Categorizing emails as spam or not.
- Video Annotation: Tracking moving objects in a video frame by frame.
The Importance of Data Annotation in AI and Machine Learning
Without Data Annotation Tech, AI models would be blind to context. Machine learning algorithms rely on labeled data to learn the differences between a cat and a dog in images, for instance. Annotation allows machines to learn from past data and make predictions about future data.
Statistics:
- According to a recent study, 80% of AI model failures are due to poor data quality, which highlights the critical importance of data annotation in ensuring accurate model training.
- By 2025, the global data annotation market is expected to reach $6.45 billion, showing the increasing demand for this technology.
Tip: A high-quality annotation system can help prevent costly errors in AI model predictions.
Types of Data Annotation Tech
There are several types of data annotation methods, each suited to different kinds of machine learning tasks:
- Image Annotation: Used for tasks like object detection and facial recognition.
- Text Annotation: Includes tasks like sentiment analysis, part-of-speech tagging, and entity recognition.
- Audio Annotation: Involves labeling audio data for tasks like speech recognition or emotion detection.
- Video Annotation: Combines the complexities of image and time-series annotations, often used for self-driving car algorithms and surveillance systems.
Each type of annotation has unique challenges and tools, but all contribute to improving AI model performance.
Manual vs. Automated Data Annotation
Data Annotation can be done manually or automatically. In manual annotation, humans carefully label data, ensuring high accuracy, while in automated annotation, machine learning models perform the task, improving speed and scalability but potentially sacrificing precision.
Key Difference: Automated annotation is increasingly popular as datasets grow larger. However, human annotators are still essential for complex or nuanced tasks where context is key.
Method | Pros | Cons |
---|---|---|
Manual | High accuracy, contextual | Time-consuming, expensive |
Automated | Fast, scalable | Lower accuracy, needs human oversight |
How Data Annotation Tech Enhances AI Applications
From self-driving cars to virtual assistants like Siri, Data Annotation Tech plays a pivotal role in refining AI systems. Annotations make it possible for AI to recognize speech patterns, understand commands, or even predict customer behaviors in e-commerce.
Recommendation: Companies that invest in robust data annotation systems are more likely to develop successful AI products that meet customer needs.
Tools and Platforms for Data Annotation
Several tools and platforms simplify the process of data annotation, providing companies with scalable solutions:
- Amazon SageMaker Ground Truth: A popular platform for automating the data labeling process.
- Labelbox: A versatile tool that integrates human and automated labeling for various data types.
- SuperAnnotate: A tool that specializes in computer vision and image annotation tasks.
These platforms offer both manual and automated solutions, allowing businesses to choose the approach that best suits their needs.
Challenges in Data Annotation Tech
Despite its importance, Data Annotation Tech faces several challenges:
- Scalability: As datasets grow larger, the need for more efficient annotation techniques becomes urgent.
- Cost: High-quality manual annotations can be expensive, especially for niche tasks.
- Bias: Annotator biases can influence the labeling process, leading to skewed AI predictions.
Solution:
One approach to addressing these challenges is to combine automated annotation with human oversight, ensuring that large datasets can be annotated quickly without sacrificing quality.
Future Trends in Data Annotation Tech
The future of Data Annotation Tech is likely to be shaped by increasing automation and advancements in AI-assisted annotation. Automated systems will evolve to handle more complex tasks, reducing the reliance on human annotators.
Emerging Trend: Active learning, a method where the machine learning model actively selects the most useful data to annotate, is gaining traction as a way to improve annotation efficiency.
FAQs
What industries benefit most from Data Annotation Tech?
Industries like healthcare, automotive, finance, and retail all rely on annotated data for AI applications ranging from medical imaging to fraud detection.
Is automated data annotation reliable?
While automated annotation is fast, it often requires human oversight to ensure accuracy, particularly for complex tasks.
How can businesses reduce data annotation costs?
By combining automated tools with manual checks and focusing on high-value annotations, businesses can optimize both cost and quality.
Conclusion
Data Annotation Tech is the backbone of AI and machine learning, driving innovation in industries ranging from healthcare to e-commerce. As businesses and AI applications scale, the need for accurate, efficient, and cost-effective data annotation continues to grow.
Are you using Data Annotation Tech in your business? How has it impacted your AI solutions? Leave a comment below and explore our other blogs to learn more about the future of AI and machine learning.